Data downtime is a critical challenge in the modern business landscape that can silently erode an organization's operational efficiency and bottom line.
Data downtime refers to periods when data is incomplete, inaccessible, inaccurate, or entirely unavailable, preventing businesses from making timely and informed decisions.
It's the digital equivalent of a manufacturing plant suddenly grinding to a halt – except in this case, the stoppage happens in your data pipelines, analytics systems, and critical business intelligence platforms.
The Initial Challenge:
A Fortune 500 retailer facing a complex and increasingly problematic data reliability issue. With operations spanning thousands of stores, multiple online platforms, and an intricate supply chain, the company was experiencing frequent and costly data interruptions that were impacting every aspect of their business.
The key challenges they encountered included:
- Inconsistent Data Integrity: Multiple data sources were producing conflicting information, making it difficult to trust any single source. Inventory reports, sales analytics, and customer insights were often misaligned, leading to potential millions in misguided business decisions.
- Unpredictable Data Pipeline Failures: Their existing data infrastructure was prone to unexpected breakdowns. On average, they were experiencing 15-20 hours of data downtime per month, which translated to significant operational disruptions.
- Complex Data Ecosystem: With data flowing from POS systems, e-commerce platforms, inventory management tools, and CRM systems, tracking and maintaining data quality had become increasingly complex.
The Strategic Approach to Tackle Data Downtime
Comprehensive Data Observability
The journey began with implementing a robust data observability platform that significantly enhanced their data management capabilities. This system provided real-time monitoring of data quality, enabling instant detection and response to anomalies. It also allowed for detailed lineage tracking, giving teams precise visibility into each data point's journey through complex systems.
Advanced Data Validation Frameworks
Building on their observability foundation, the team developed advanced data validation mechanisms that meticulously examined data completeness at each pipeline stage. Automated quality checks identified potential issues before impacting operations, while robust fallback mechanisms ensured resilience against total data failure.
Redundant Data Infrastructure
To ensure true data reliability, the retailer invested in a redundant infrastructure capable of withstanding challenges. They established multiple backup systems and geo-distributed storage solutions to maintain data integrity across locations. Automatic failover mechanisms ensured seamless transitions during any disruptions.
Machine Learning-Driven Predictive Maintenance
The integration of machine learning algorithms transformed their data management strategy. These systems predicted potential pipeline failures accurately, shifting from reactive problem-solving to proactive prevention. They could automatically resolve minor issues and provide recommendations for infrastructure improvements, turning data management into a strategic advantage.
.png)
The Shift to Observability in Modern Stack
The shift to data observability represented more than just a technological upgrade – it was a fundamental transformation in how the organization approached data management.
Modern technology environments are no longer simple, linear systems. They're complex, interconnected networks of microservices, cloud platforms, distributed systems, and dynamic data pipelines. According to a BDAN survey approximately 2.5 quintillion bytes of data are generated each day, equating to about 328.77 million terabytes or 0.33 zettabytes daily
Traditional monitoring tools, which act as basic temperature checks, are insufficient for managing such vast and intricate environments. Observability platforms like Datachecks offer features like continuous data monitoring, automated anomaly detection, data replication testing and other for modern stacks with end-to-end visibility. This comprehensive observability ecosystem transforming how organizations understand and manage their data, maintaining stable performance and reliability.
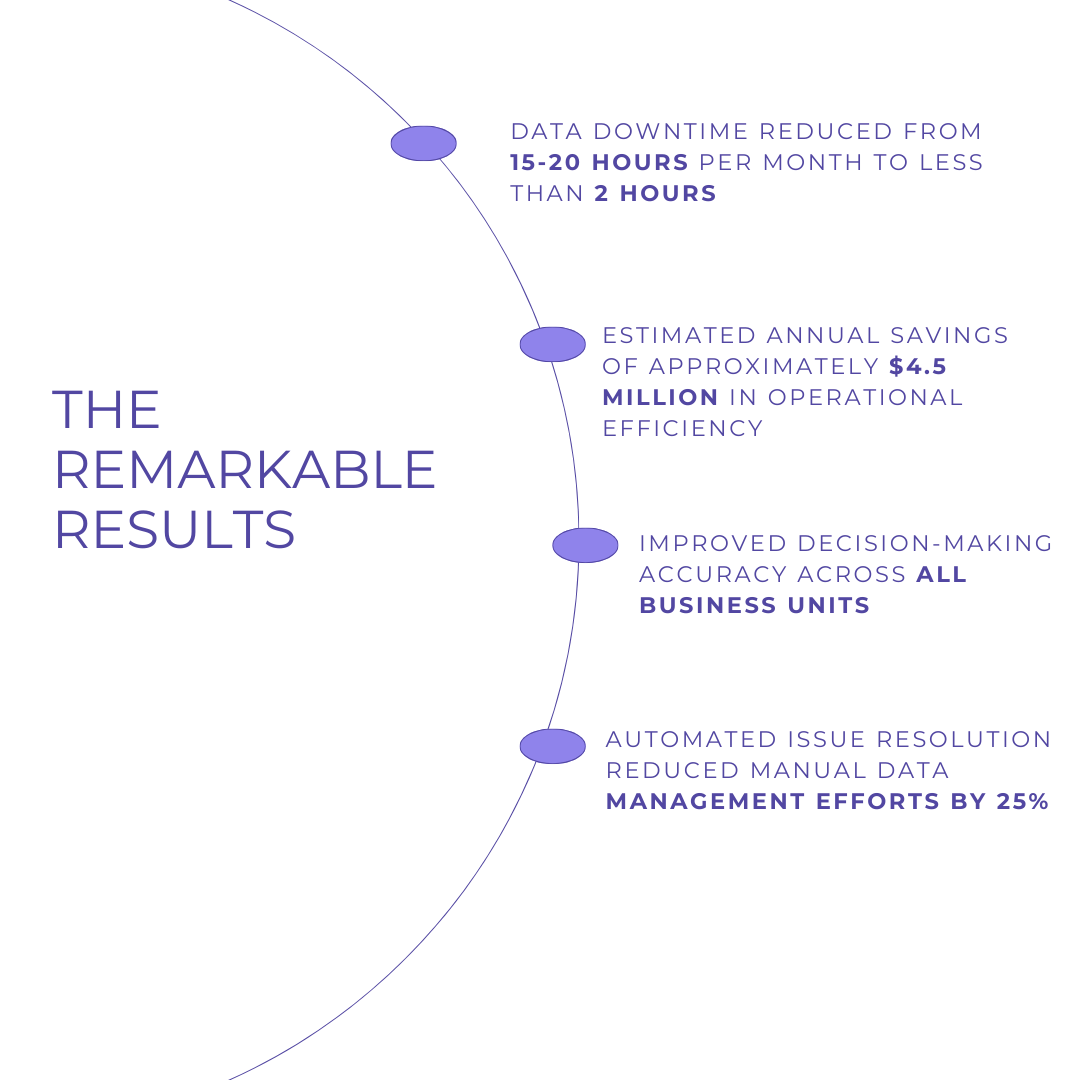
Looking Forward
Data downtime is no longer an isolated inconvenience; it is a significant business risk that can undermine decision-making, disrupt operations, and erode customer trust. The Fortune 500 retailer's journey from frequent data disruptions to a highly resilient data ecosystem underscores the transformative power of modern data observability, validation frameworks, and machine learning-driven predictive maintenance.
As the data landscape continues to evolve, more industry giants are recognizing the critical need for robust data management strategies. Companies such as Google, Microsoft, Amazon, Netflix, Uber and other are investing heavily in data observability and reliability frameworks to ensure seamless data flow across their complex infrastructures.
In the data-driven economy, maintaining data quality and uptime is not just a technical challenge but a strategic imperative.
Want to stay ahead in the world of data quality and observability? Subscribe to the Datachecks Newsletter and join a community of forward-thinking professionals. Get the latest insights, best practices, and exclusive updates delivered straight to your inbox. Sign up now!