Predictive models have become the backbone of strategic decision-making across industries. However, the old adage "garbage in, garbage out" has never been more relevant. The accuracy and reliability of predictive analytics fundamentally depend on the quality of data feeding these sophisticated algorithms.
Predictive models are the cornerstone of forward-thinking innovation, driving insights that shape the future of industries. Yet, their success hinges on a simple truth: Flawed data leads to flawed outcomes. No matter how advanced the algorithms, the integrity of the predictions rests entirely on the quality, consistency, and completeness of the data fueling them.
Data Quality and Its Role in Predictive Analytics
Predictive analytics transforms raw data into actionable insights, enabling organizations to forecast trends, mitigate risks, and optimize performance. However, this powerful capability is only as strong as the data foundation it relies upon. Data quality represents the cornerstone of effective predictive modeling, encompassing multiple critical attributes that determine the model's potential for generating meaningful and reliable predictions.
The Impact of Poor Data Quality
When data quality is compromised, the consequences can be profound and far-reaching:
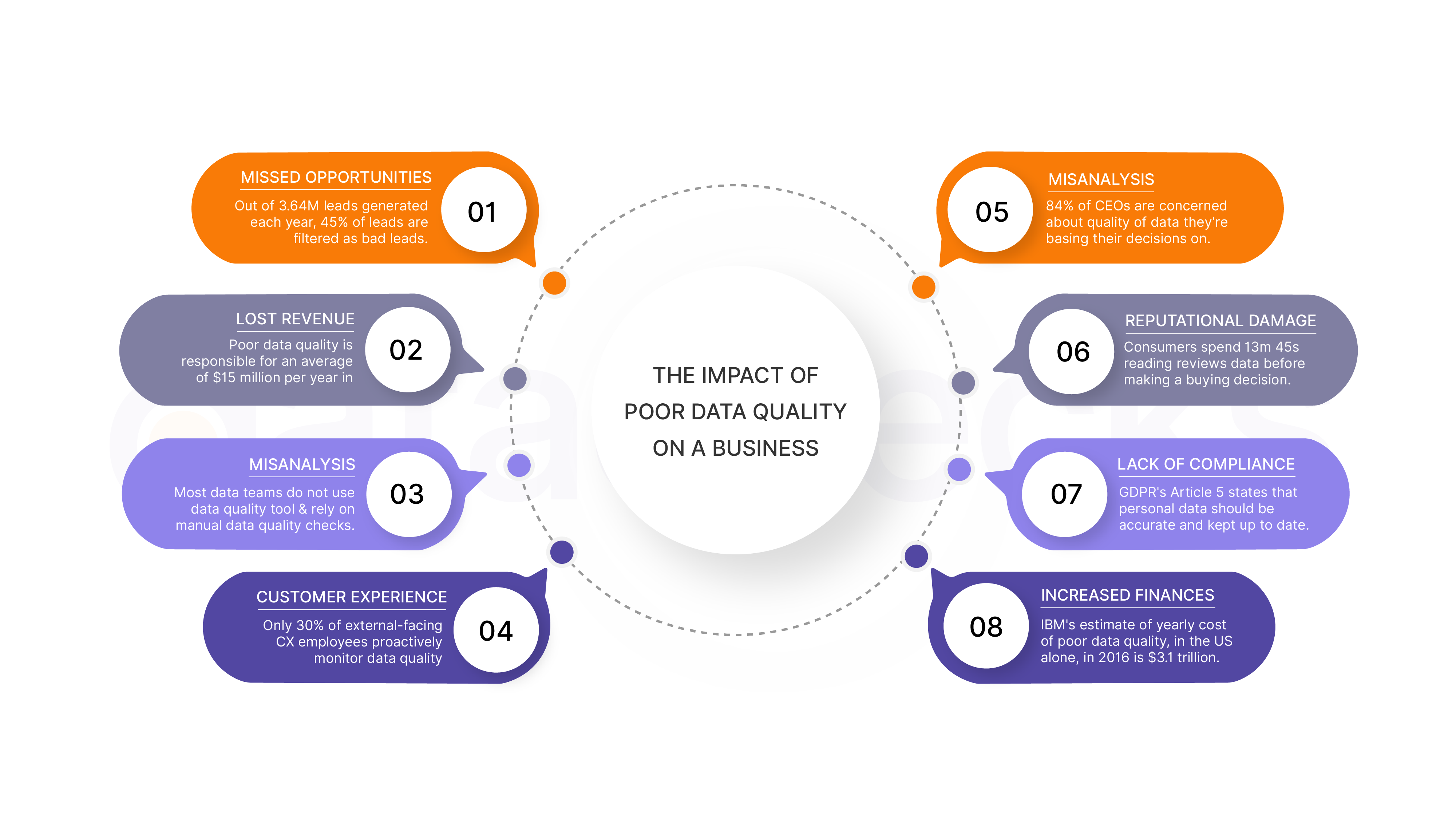
According to Gartner research, poor data quality can cost organizations an average of $12.9 million per year. This includes the cost of wasted resources on data cleaning, inaccurate analysis leading to bad decisions, and missed opportunities due to unreliable insights
Key Dimensions of Data Quality:
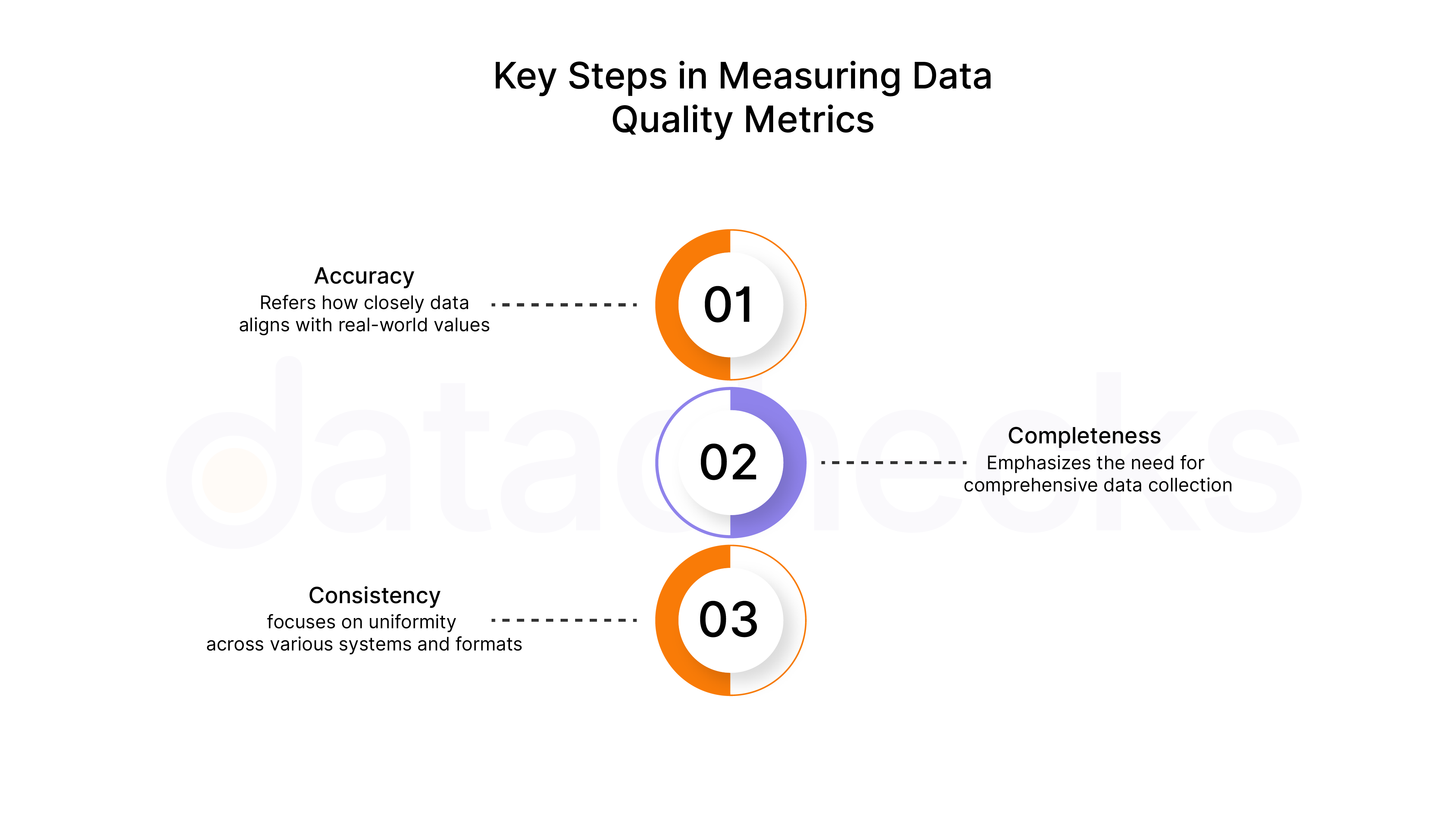
The key dimensions of data quality can be categorized into three main areas:
Accuracy is the most critical dimension of data quality it refers how closely data aligns with real-world values. In predictive analytics, accuracy is not just a technical requirement; it's a strategic necessity. Inaccurate data can turn predictive models into sources of misguided decisions, leading organizations to inefficiencies and wasted resources.
Completeness emphasizes the need for comprehensive data collection. A dataset with missing information resembles a puzzle with essential pieces absent. Such gaps can distort analytical outcomes and introduce biases, undermining the reliability of predictions. Organizations should ensure that all relevant data points are captured to support robust analysis.
Consistency focuses on uniformity across various systems and formats. In today's complex technological landscape, maintaining consistent data representation is vital to prevent misinterpretations and reduce confusion. Consistent data serves as a universal language, facilitating effective communication and analysis across different areas of an organization.
Best Practices for Maintaining High Data Quality Standards
Implement Data Governance Frameworks
Establishing clear policies and standards for data collection, storage, and management is crucial for maintaining high data quality. A well-defined governance framework ensures that all data-related activities align with organizational objectives and regulatory requirements. Additionally, creating cross-functional teams responsible for data quality oversight fosters collaboration across departments which enables a more holistic approach to data management.
Utilize Advanced Data Cleaning Technologies
Leveraging machine learning and AI-powered data cleansing tools can significantly enhance the quality of your datasets. These advanced technologies automate the process of identifying and rectifying errors, ensuring that data remains accurate and reliable. Furthermore, developing automated validation and correction mechanisms can help organizations maintain high data integrity over time, reducing the manual effort required for data cleaning.
Regular Data Audits and Monitoring
Conducting periodic comprehensive data quality assessments is essential to identify potential issues before they escalate. Regular audits help organizations track data quality metrics and performance indicators, providing insights into areas that require improvement. By implementing a systematic monitoring process, organizations can ensure ongoing compliance with established data quality standards.
The Role of Data Observability Platforms
Modern data observability platforms Datachecks leverage advanced machine learning and artificial intelligence to create dynamic data quality management systems. These platforms can automatically detect anomalies, track data lineage, and provide comprehensive insights into data performance. By offering end-to-end visibility across complex data infrastructures, they enable organizations to maintain high-quality data standards with unprecedented precision and efficiency.
Data observability platforms excel in addressing these dimensions by providing:Real-time anomaly detection that immediately flags potential accuracy issuesComprehensive data lineage tracking to ensure completenessCross-system consistency checks that validate data representation across different platforms
The Fortune 500-Way
Many Fortune 500 companies are leveraging predictive analytics to optimize their operations and enhance customer experiences through data-driven strategies.
Amazon integrated predictive analytics to refine its inventory management and supply chain operations. This approach focuses on ensuring data accuracy and completeness, which has significantly reduced instances of stockouts by approximately 30% and overstock situations by about 25%. As a result, operational efficiency has improved with fulfillment speeds increasing by up to 20% during peak demand periods.
Walmart utilizes advanced predictive models to forecast demand across its extensive network of stores. By prioritizing data consistency and accuracy, the company has successfully minimized waste and optimized logistics. This strategic focus has resulted in substantial savings in operational costs. For instance, Walmart's recent implementation of GPU-based demand forecasting has enhanced forecast accuracy by 1.7% compared to previous models, allowing for more precise predictions for approximately 100,000 products across its 4,700 stores in the U.S.
Target's application of predictive analytics extends to personalizing its marketing campaigns. By analyzing high-quality customer data, Target is able to tailor promotions to specific demographics. This targeted approach has led to increased conversion rates and strengthened customer loyalty.
Looking forward
Organizations must embrace a holistic approach to data management. This involves developing robust governance frameworks, investing in advanced cleaning technologies, conducting regular audits, and cultivating a culture that values data as a strategic resource. The most successful companies will be those that view data quality not as a periodic task but as an ongoing, organization-wide commitment.
Data is no longer just a byproduct of business operations; it is a strategic resource that demands meticulous management and continuous optimization. The emergence of advanced technologies like data observability platforms represents a transformative approach to data quality management. These sophisticated tools offer unprecedented visibility into data ecosystems, enabling organizations to proactively identify, diagnose, and resolve potential issues before they cascade into significant strategic missteps.
Subscribe to the Datachecks Newsletter and join a community of forward-thinking professionals. Get the latest insights, best practices, and exclusive updates delivered straight to your inbox. Sign up now!