As businesses increasingly depend on automated decision-making systems for operational insights and decision-making, the need for high-quality data becomes critical. These systems are driven by artificial intelligence (AI) and machine learning (ML) algorithms, which rely on vast amounts of data to function effectively.
Inaccurate, incomplete, or biased data can significantly skew the decisions made by these systems. For instance, if input data is flawed, it can lead to suboptimal outcomes, compromising the effectiveness of automated decisions. Good data quality not only ensures accurate decision outcomes but also boosts operational efficiency, saving both time and resources for businesses.
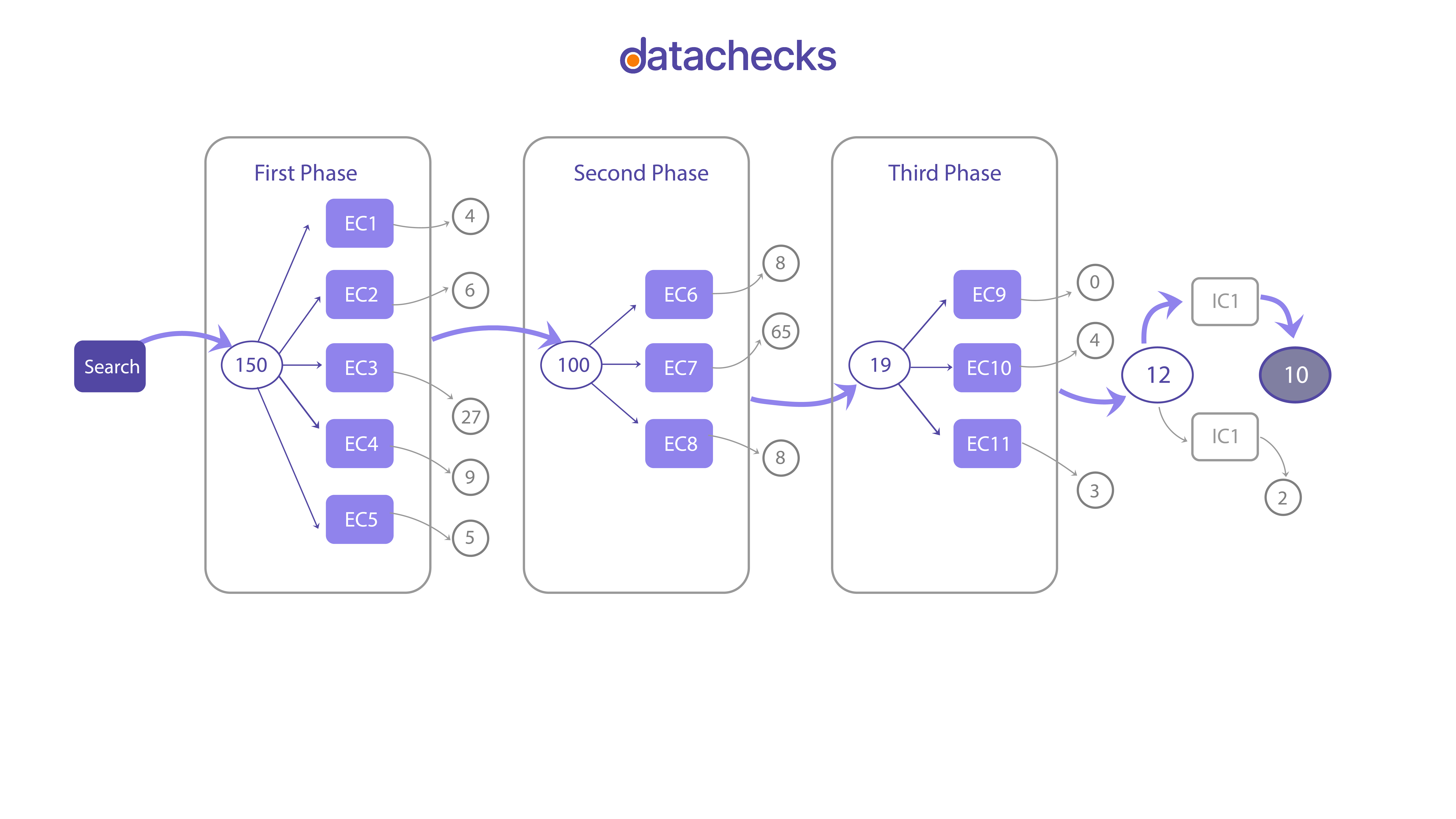
Challenges in Maintaining Data Quality
Data quality issues such as inaccuracies, incompleteness, and biases can result from a variety of sources. These challenges are further magnified in automated systems, where the volume of data and speed of decision-making make manual checks impractical. Without consistent monitoring and validation protocols, businesses risk compromised decisions and missed opportunities.
To address these challenges, organizations must implement robust data governance frameworks and utilize continuous monitoring systems. This proactive approach ensures that the data feeding into automated systems is accurate and reliable, enhancing the trustworthiness of decision outcomes.
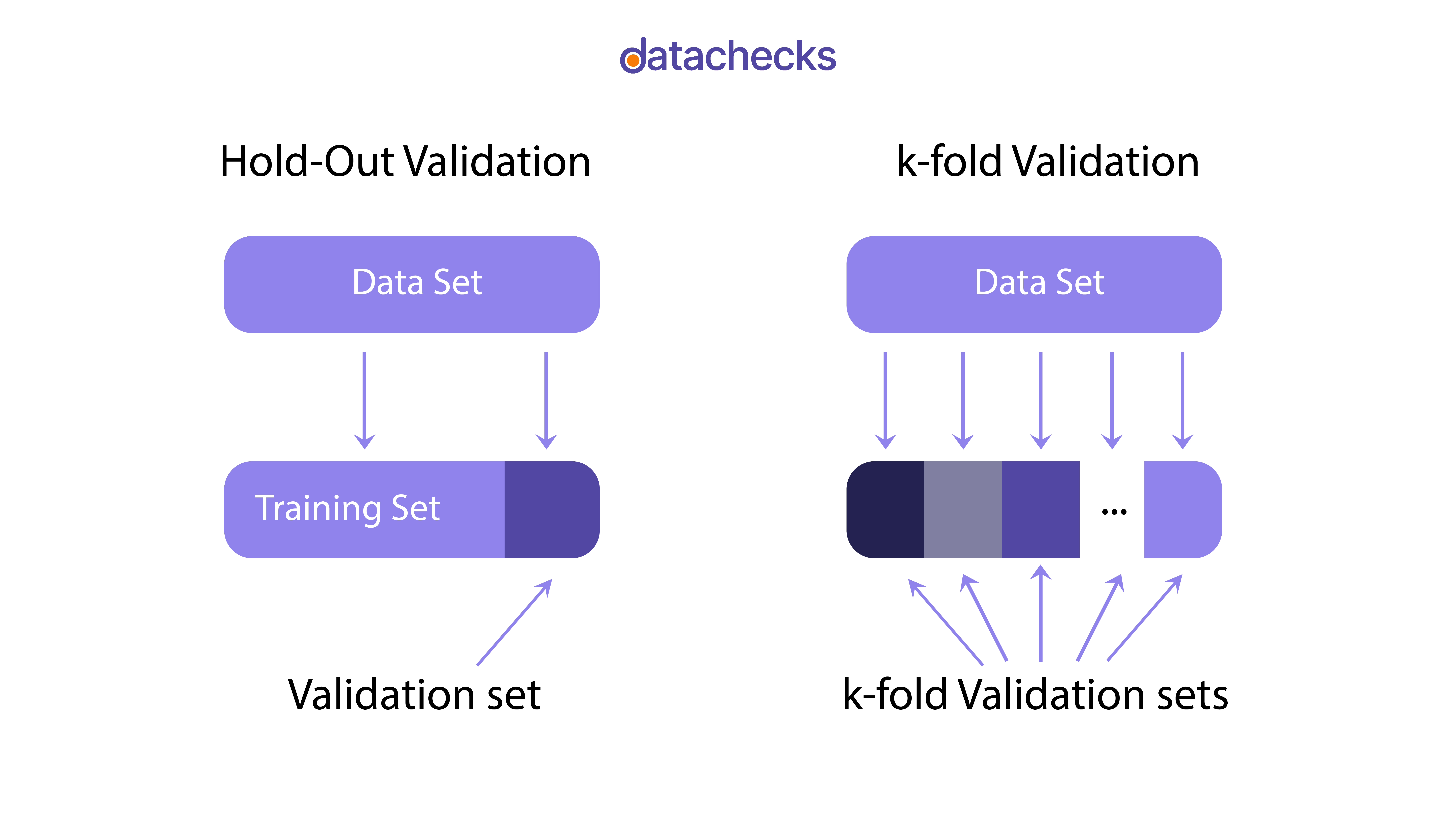
Impact on Decision-Making and Operational Efficiency
The relationship between data quality and decision accuracy is profound. As organizations scale, the complexity of data increases, making it harder to maintain data integrity. According to the paper, companies with robust data quality practices experience improved decision-making, operational efficiency, and cost-effectiveness. Clean, reliable data helps businesses make informed choices faster and with greater confidence.
Mitigating Biases and Ensuring Ethical Decision-Making
One significant aspect of data quality is its impact on ethical decision-making. Automated decision systems can inadvertently reinforce biases present in the data, leading to unfair or discriminatory outcomes. To mitigate this, businesses must prioritize data quality management alongside ethical guidelines, ensuring fairness and transparency in decisions made by AI and ML systems.
Proactive Data Governance: A Key to Success
The key takeaway from the paper is the importance of data governance. Organizations must adopt comprehensive frameworks to regulate data quality processes, ensure accountability, and continually audit data flows. This governance helps maintain data integrity, thereby enhancing the reliability of automated decision-making systems.
In conclusion, data quality is not just a technical requirement—it is foundational to the success of automated decision-making systems. Businesses that invest in data quality frameworks, ethical decision-making, and continuous monitoring will have a significant advantage in the continuously evolving digital landscape.