As the banking sector evolves, the demand for high-quality data has never been more critical.Infact, a recent foresster research advices that 'Data quality is the primary factor limiting GenAI adoption for most B2B businesses'.
Data quality automation is set to revolutionize how banks manage their data, ensuring compliance, enhancing decision-making, and improving customer experiences. This blog explores the future of data quality automation in banking, highlighting key trends, technologies, and best practices.
The Importance of Data Quality in Banking
Data quality is foundational to effective banking operations. Poor data quality can lead to significant financial repercussions and compliance failures. For example, HSBC was fined over $1.9 billion due to inadequate data management practices related to anti-money laundering (AML) compliance. According to a recent study by Gartner, more than 30% of the GenAI projects will fail due to failed POC by end of 2025.
Key Trends Shaping Data Quality Automation
- Artificial Intelligence and Machine Learning: AI and ML technologies are at the forefront of data quality automation. These tools can identify anomalies, predict potential violations, and initiate corrective actions without manual intervention. Dr. Michael Stonebraker, a leading expert in database systems, emphasizes that "the future of data management lies in automating the mundane tasks that consume valuable resources."
- Self-Service Data Quality Solutions: A shift towards self-service data quality tools empowers business users to proactively identify and resolve data issues. This democratization of data management allows for quicker response times and reduces reliance on IT departments, fostering a culture of accountability across the organization.
- Integration with Data Observability Platforms: Data observability provides comprehensive visibility into data pipelines, enabling banks to monitor the flow of information in real-time. According to a report by McKinsey & Company, organizations that adopt observability practices can reduce data-related incidents by up to 50%.
Best Practices for Implementing Data Quality Automation
- Establish Clear Governance Frameworks
Implementing robust data governance frameworks is essential for maintaining high data quality standards. This includes defining roles and responsibilities for data stewardship and establishing protocols for data entry and validation. - Utilize Domain-Specific Solutions
Many existing data quality tools lack the specificity required for banking operations. Purpose-built solutions that incorporate domain knowledge can address unique challenges faced by financial institutions, such as compliance-specific failure modes. - Invest in Continuous Monitoring Systems
Continuous monitoring systems allow banks to maintain oversight of their data quality in real-time. By leveraging adaptive rules powered by machine learning, these systems can automatically alert stakeholders to potential issues, facilitating timely interventions.
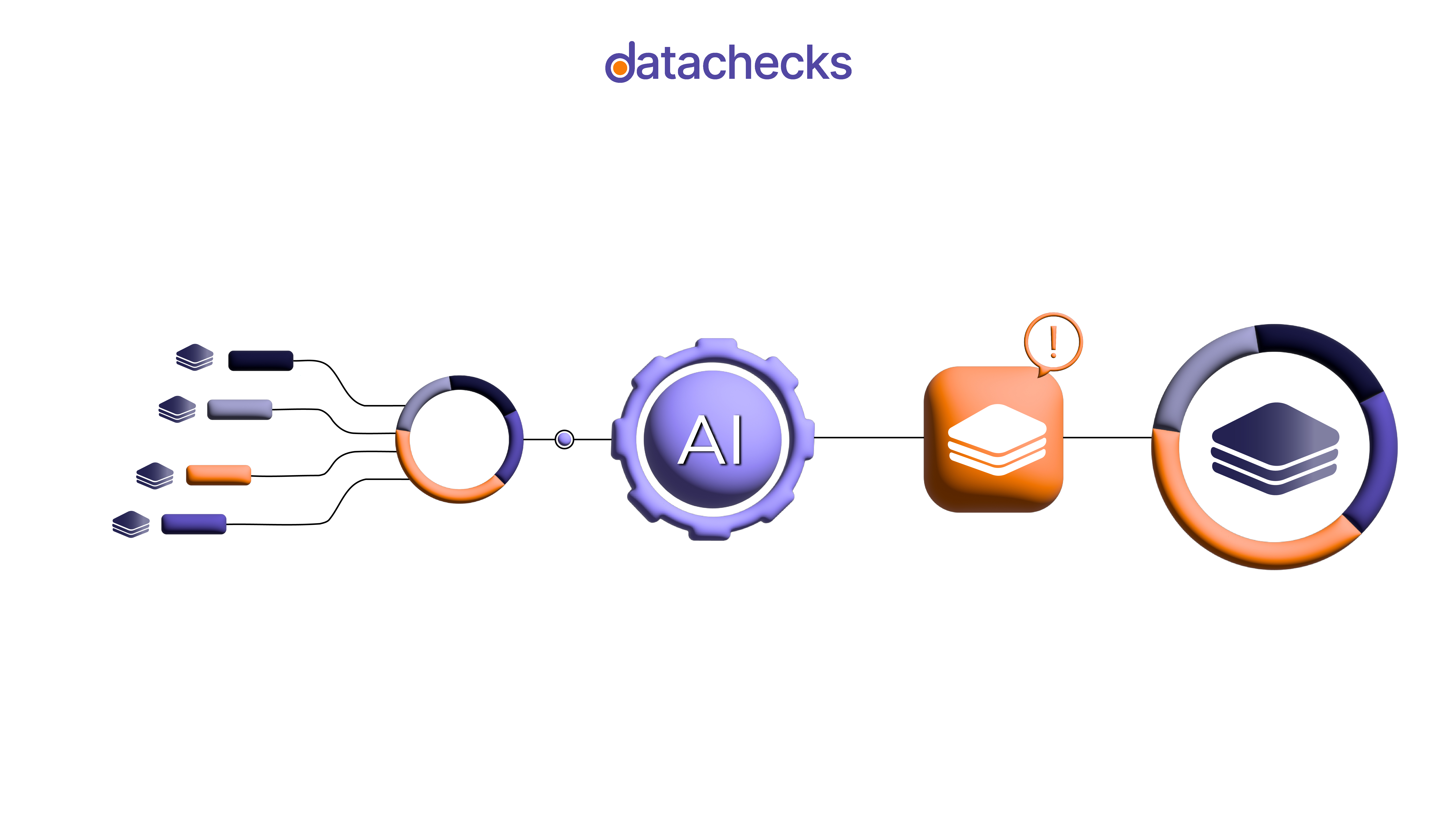
Data Quality Automation Architecture
Conclusion
The future of data quality automation in banking is bright, driven by advancements in AI, machine learning, and self-service solutions. By adopting these technologies and best practices, banks can ensure high-quality data that supports compliance, enhances decision-making, and improves customer experiences. As Dr. Claudia Imhoff states, "In the era of big data, organizations must prioritize their data quality strategies or risk falling behind their competitors."