Organizations rely on accurate, timely, and actionable data to make strategic decisions, optimize operations, and stay ahead of the competition. However, what happens when data is unreliable or bad? Poor data quality can lead to significant hidden costs, causing ripple effects throughout an organization and affecting everything from customer satisfaction to revenue generation.
Common Types of Bad Data
Companies encounter bad data in many forms—such as inaccurate, incomplete, or unreliable information—that can negatively impact decision-making.
- Incomplete Data: Data that lacks essential information, fields, or attributes. This will skew analysis and lead to incorrect conclusions.
- Duplicate Data: Data that is repeated unnecessarily. This inflates metrics, increases storage costs, and causes discrepancies in analysis.
- Inconsistent Data: Data that has conflicting values across different systems or databases. This leads to confusion and inaccurate results.
- Outdated Data: Data that is no longer relevant or accurate due to changes over time. Using outdated data will result in misleading decisions.
- Incorrect Data: Data that contains errors such as typos, misclassifications, or inaccurate values. This compromises the integrity of any analysis.
- Invalid Data: Data that doesn't adhere to the correct format, range, or validation rules. This can prevent the data from being used effectively in analysis or operations.
According to a Harvard Business Review, only 3% of enterprise data is deemed to be of acceptable quality (scoring 97% or higher), while 15% is considered mostly accurate (scoring 80% or higher). The unfortunate truth is that data teams will inevitably encounter bad data at some point.
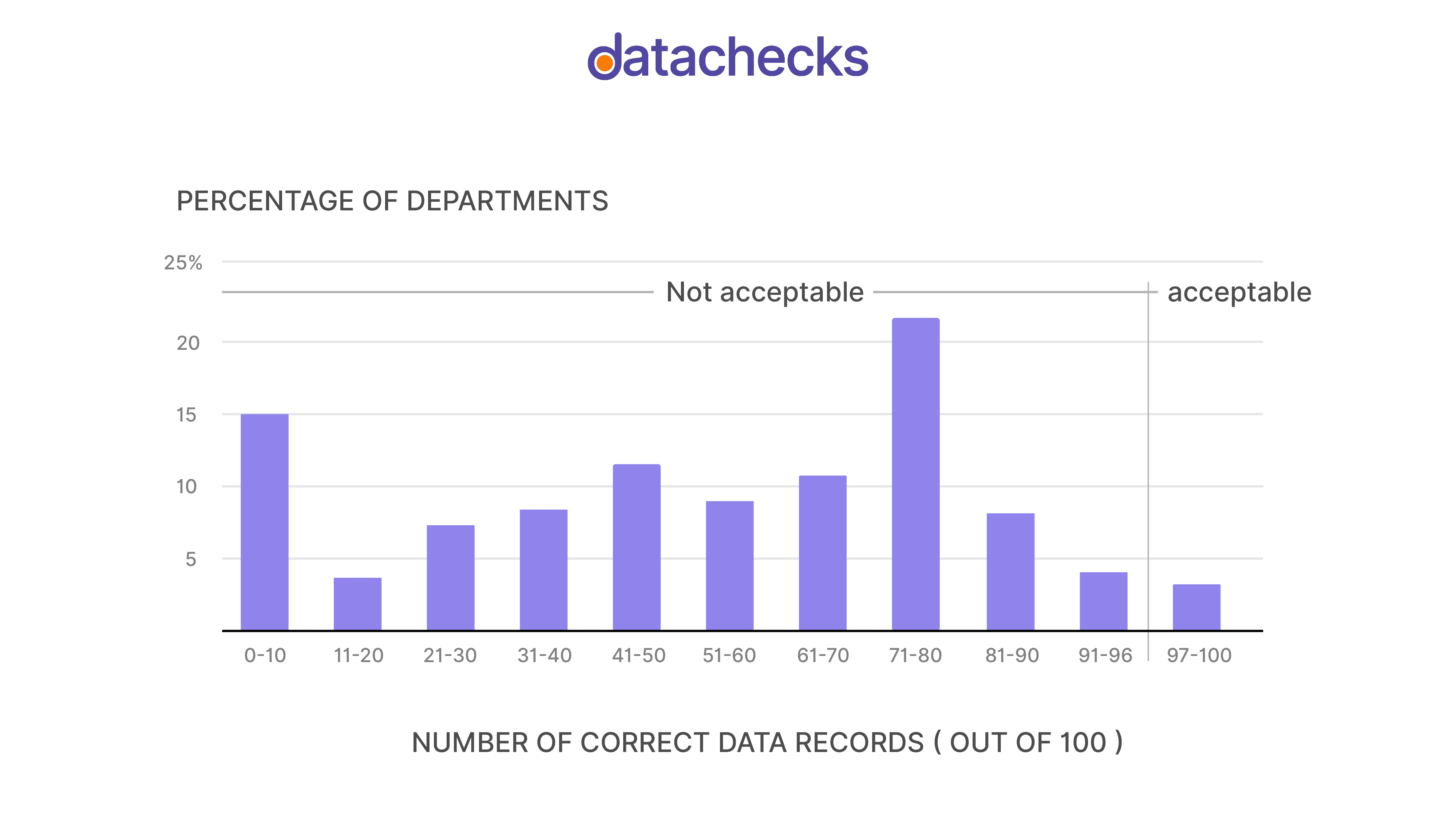
The Hidden Cost of Bad Data
There is a well-known idea in software development often called the '1-10-100 cost rule,' illustrating how the cost of fixing an issue multiplies based on when it's caught. In the earliest stages, such as development, addressing a problem might cost $1. However, if that issue is found during testing or staging, the cost could jump to $10. If it remains undetected until production, resolving it may cost $100.
The same idea holds true for data processes. When errors are identified and corrected at the data ingestion stage, the cost impact is relatively low. If they are discovered during data transformation, the cost grows significantly. And if these issues reach the end-user level without correction, the cost to address them can escalate drastically, highlighting the importance of early detection and quality control.
According to a recent survey of over 1,000+ data pipelines, 72% of data quality issues were found after the impact had already occurred, and 40% of data team time was lost troubleshooting these issues.

How to Avoid the Impact of Bad Data
The key to preventing bad data issues lies in implementing continuous monitoring, detection, and resolution processes. Organizations must establish robust systems for continuously monitoring data quality, detecting issues early in the pipeline, alerting teams to data incidents, and resolving problems before they become major concerns.
Data observability platforms like Datachecks automate the entire process, detecting data quality issues in complex data pipelines before they become major problems. You can continuously monitor your data health, alert teams when issues arise, and maintain confidence in your data.
Taking Action
To improve data reliability and avoid bad data issues, organizations should implement automated data quality monitoring solutions. These systems enable continuous data health monitoring, timely team alerts, and enhanced confidence in data quality. They also reduce troubleshooting time and prevent costly data-related mistakes.
By prioritizing data quality and implementing proper monitoring systems, organizations can significantly reduce the hidden costs associated with bad data and make more informed, data-driven decisions.
Improve your data reliability and avoid bad data with Datacheks.