Organizations are increasingly reliant on data pipelines to power their decision-making processes. Our analysis of over 1,000 data pipelines across various industries reveals critical insights into the current state of data quality and the challenges organizations face in 2024.
Poor data quality puts organizations at significant risk, as problems cascade through downstream systems and affect critical business operations before being detected. From the analysis, concerning trends have emerged:
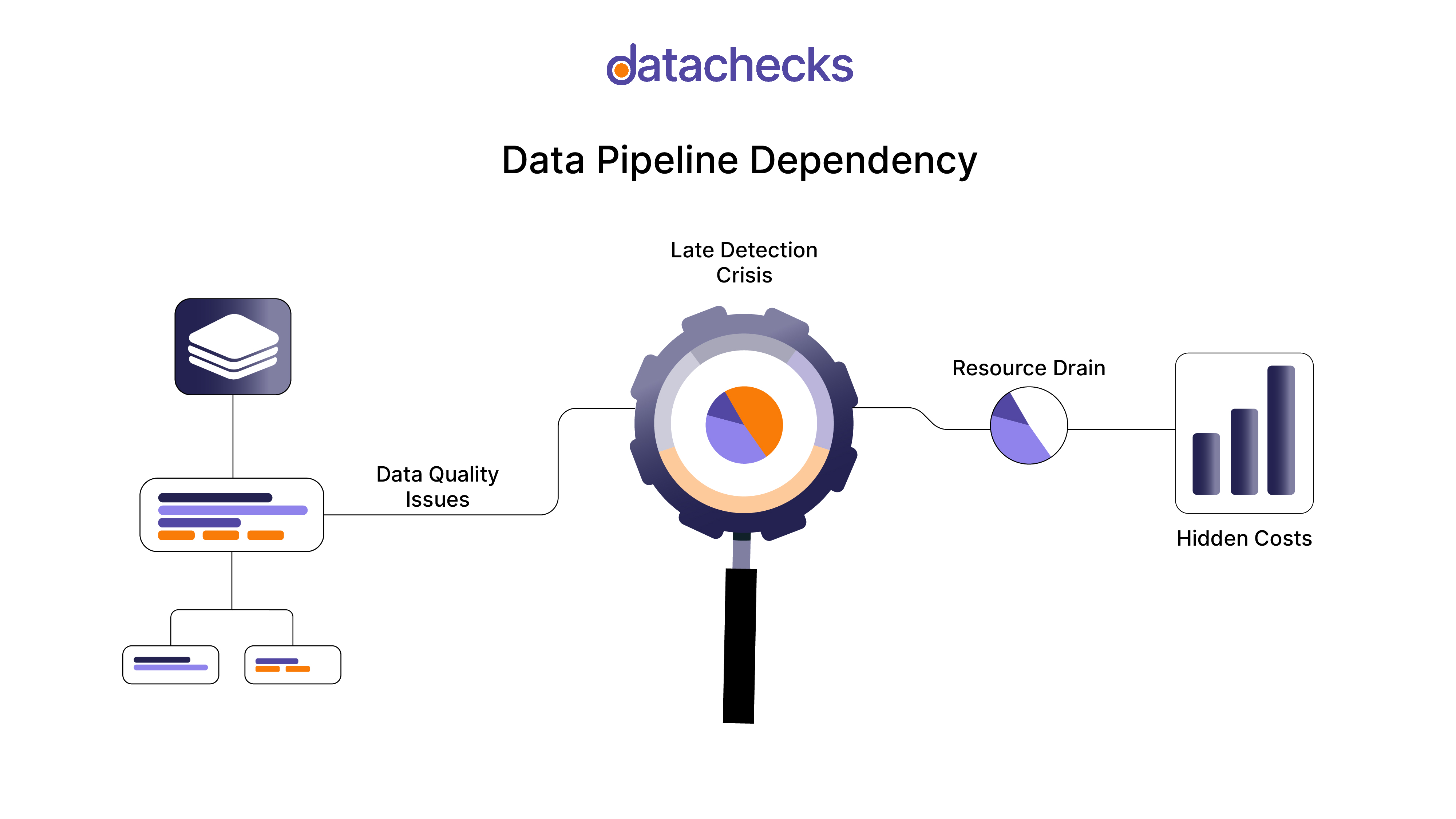
Late Detection Crisis: 72% of Data Quality Issues Found Post-Impact
Data quality issues lurk silently until they surface through their consequences. Our analysis reveals that 72% of these issues are discovered only after they've already affected business decisions. This late detection pattern means errors propagate through systems, compound their impact, and create cascading effects before anyone notices.
Key Metrics:
- Average time to detect: 12.3 days
- Downstream systems affected: 4.7 per incident
- Impact severity: 64% critical or high
- Average resolution time after detection: 48 hours
- Data issues affecting financial reports: 43%
Resource Drain: 40% of Data Team Time Lost to Troubleshooting
In a concerning trend, data teams are spending nearly half their working hours on problem resolution rather than value creation. This 40% time investment in troubleshooting represents a massive drain on specialized talent, diverting skilled professionals from strategic initiatives to constant firefighting mode.
Key Metrics:
- Weekly hours spent debugging: 16.2
- Delayed project deliveries: 57%
- Unplanned work allocation: 35%
- Cross-team coordination time: 12 hours/week
- Documentation backlog: 68%
Hidden Costs: 30% of Data Quality Issues Lead to Revenue Loss
Data quality issues often remain concealed until they manifest as significant financial Consequences. Analysis indicates that 30% of these issues result in direct revenue loss, affecting the bottom line and strategic growth initiatives. This hidden cost underscores the need for proactive data monitoring to mitigate potential risks before they escalate.
Key Metrics:
- Estimated revenue loss per incident: $200,000
- Percentage of organizations experiencing revenue loss: 30%
- Average time to identify revenue-impacting issues: 15 days
- Frequency of revenue loss incidents: 2.3 per quarter
- Impact on customer trust and retention: 50% decline in satisfaction
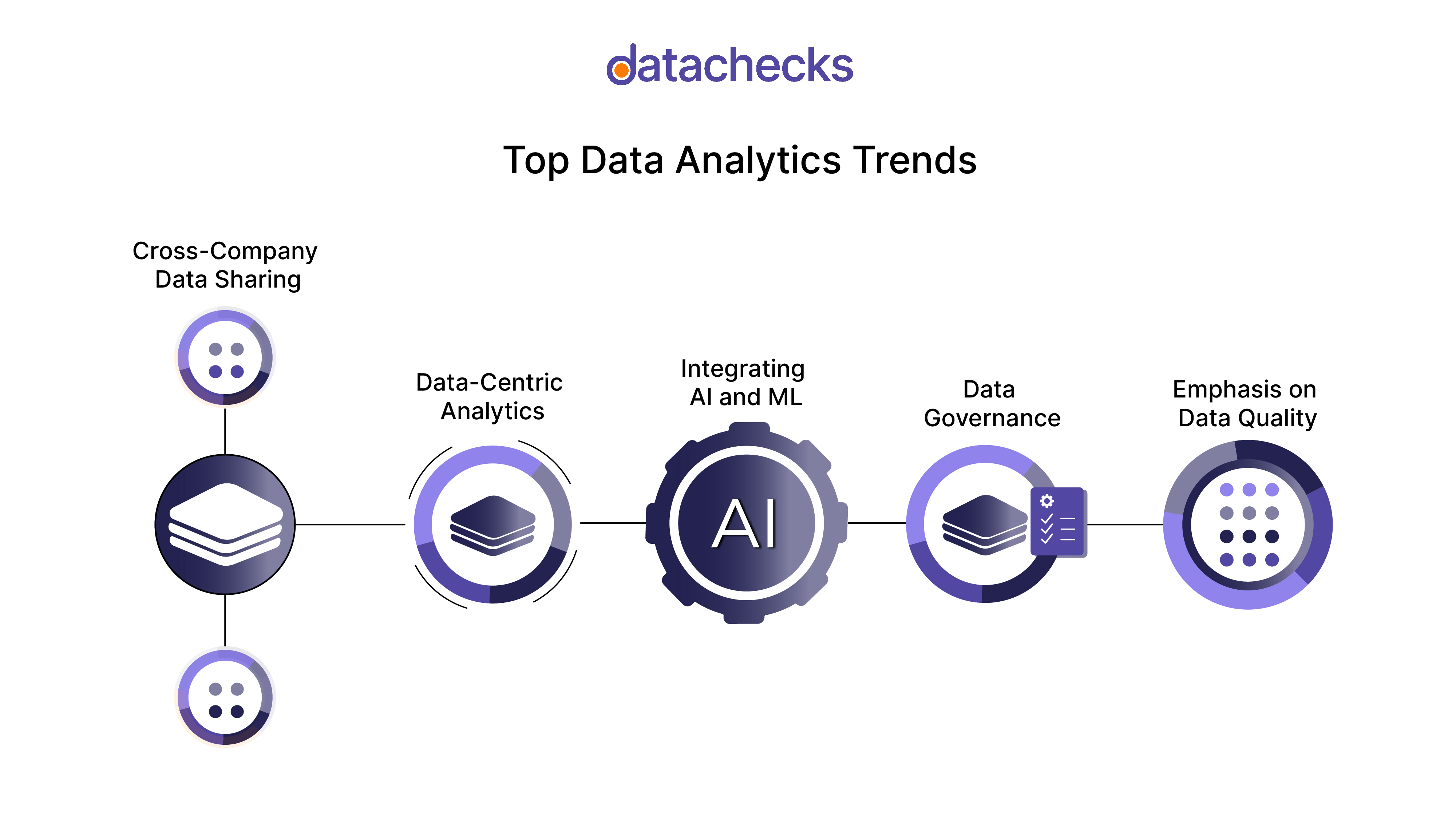
How Data Observability Platforms Address These Challenges:
However, there's a clear path, Data Monitoring. But relying on traditional approaches that involve manually monitoring data is not only difficult but also wastes valuable human hours and is prone to errors. To address these issues, data observability platforms like Datachecks automate the entire process, providing end-to-end visibility into data pipelines.
Let’s explore some real-world examples of how leading companies are utilizing data observability to overcome common data challenges:
DoorDash: How DoorDash Maintains Real-time Delivery Data Accuracy
Challenge:
DoorDash processes millions of real-time delivery updates every day, which is essential for efficiently assigning drivers and providing accurate delivery time estimates to customers. However, frequent data delays were affecting driver assignments, leading to inaccurate delivery time estimates and ultimately impacting customer satisfaction.
Solution Implementation:
DoorDash deployed end-to-end data monitoring across its delivery pipeline. With automated freshness checks every 5 minutes and anomaly detection specifically for delivery time predictions, DoorDash gained real-time visibility into data issues.
Results:
- An 83% reduction in data delay incidents
- A 45% improvement in delivery time accuracy
- An estimated $2.1 million in annual savings in operational costs due to fewer delays and improved efficiency.
Stripe: How Stripe Improves Payment Processing Data Quality
Challenge:
As a global payments company, Stripe processes billions of transactions daily, requiring extremely high data accuracy for financial reconciliation and compliance with complex cross-border payment rules. Any error or delay in this data could lead to inaccuracies in financial reporting or missed fraud detection, with serious implications.
Solution Implementation:
Stripe adopted an advanced data observability platform with automated quality checks on transaction data. This setup allowed Stripe to continuously monitor transaction flows and detect inconsistencies early on.
Impact:
- A 76% reduction in payment reconciliation time
- Fraud pattern detection that was 4 times faster
- 99.99% data accuracy in financial reporting, improving trust and compliance.
Netflix: How Netflix Optimizing Streaming Quality with Real-time Monitoring
Challenge:
With billions of viewing events daily, Netflix’s data pipeline must provide high accuracy in content recommendations and real-time metrics on user experience. The challenge lies in monitoring this vast data flow to ensure viewers enjoy high streaming quality and relevant recommendations without interruptions.
Transformation:
Netflix implemented automated monitoring of viewer behavior, real-time checks on content delivery quality, and predictive analytics for streaming issues to ensure smooth operations and improve user satisfaction.
Results:
- A 56% reduction in streaming quality incidents
- A 34% improvement in recommendation accuracy
- A 23% increase in viewer engagement
The Way Forward
Organizations that have implemented mature data observability practices report 67% fewer critical incidents. This dramatic reduction demonstrates that proactive monitoring and systematic quality management aren't just technical improvements - they're business imperatives that directly impact operational efficiency and decision-making reliability.
These findings highlight a critical inflection point in data management: organizations must shift from reactive problem-solving to proactive quality assurance to remain competitive in today's data-driven landscape. The cost of inaction is no longer just technical debt - it's a direct threat to business performance and growth.