It was a typical Monday morning when Sarah, the Head of Analytics at RetailCo, received an urgent call. The company’s pricing algorithm had been quietly using corrupted inventory data for the past week, causing pricing anomalies across 200+ stores. By the time they caught the issue, they were just hours away from a major promotional campaign that could have cost them $2 million in misplaced discounts.
This near-miss highlighted a critical gap in their data infrastructure — one that many modern enterprises face today: the lack of proper data observability.
RetailCo’s Journey to Data Observability
The Challenge:
RetailCo was struggling with:
- Siloed data across multiple systems
- No unified view of data quality
- Delayed detection of data issues
- Manual data quality checks
- Limited trust in data-driven decisions
The Solution:
They implemented a comprehensive data observability platform that provided:
Automated Data Quality Monitoring
- Real-time checks for data freshness, volume, and schema changes
- Automated anomaly detection
- Historical trend analysis
End-to-End Pipeline Visibility
- Complete lineage tracking
- Impact analysis
- Root cause identification
Proactive Alert System
- Custom alerting thresholds
- Smart notification routing
- Incident management integration.
The Results:
After six months of implementation:
- 75% reduction in data incident resolution time
- 90% decrease in data quality issues reaching production
- $1.5M saved in prevented pricing errors
- 60% improvement in data team productivity
Understanding Data Observability
Data observability refers to an organization’s ability to understand, monitor, and troubleshoot data systems throughout the entire data pipeline. Think of it as your data’s health monitoring system — constantly checking vital signs, detecting anomalies, and ensuring data quality at every stage of its lifecycle.
The stakes have never been higher for data quality:
- Organizations now manage 10x more data than they did five years ago
- 95% of businesses cite the need to manage unstructured data as a problem for their business
- Poor data quality costs organizations an average of $12.9 million annually
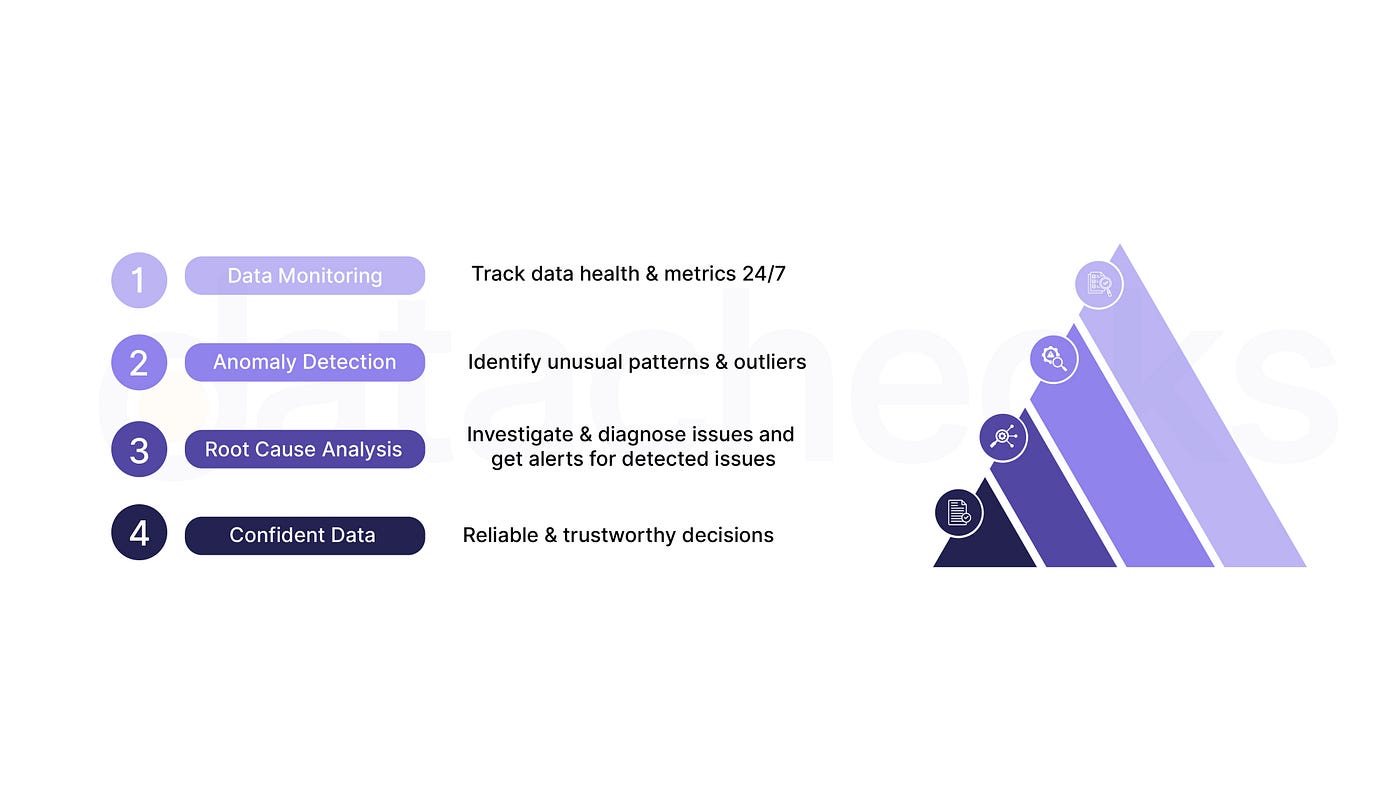
The Impact of Data Observability
The implementation of modern data observability platforms like Datachecks can have a profound impact on organizations. By providing real-time monitoring, anomaly detection, and end-to-end pipeline visibility, these platforms empower teams to proactively address data issues before they cause significant harm. As a result, RetailCo saw a 75% reduction in data incident resolution time, a 90% decrease in data quality issues reaching production, and saved $1.5 million in avoided errors.
RetailCo Before/After Data Observability
1. Inventory Management Crisis


2. Customer Loyalty Program Crisis


3. Store Performance Analytics Crisis
Before


Looking Ahead

- Start Small, Scale Fast RetailCo began with their most critical data assets and gradually expanded coverage.
- Automate Everything Manual monitoring couldn’t keep up with their data volume and velocity.
- Culture Matters Success required building a data-aware culture across teams.
As organizations continue to rely more heavily on data-driven decisions, data observability will become as fundamental as application monitoring.
RetailCo’s story demonstrates that data observability isn’t just another IT initiative — it’s a business imperative. In an age where data drives decisions, organizations can’t afford to fly blind. By implementing proper data observability practices, companies can prevent costly errors, improve efficiency, and build trust in their data-driven decisions.
The question isn’t whether to invest in data observability, but rather how quickly you can implement it before facing your own data crisis.
Subscribe to the Datachecks Newsletter and join a community of forward-thinking professionals. Get the latest insights, best practices, and exclusive updates delivered straight to your inbox. Sign Up Now!